
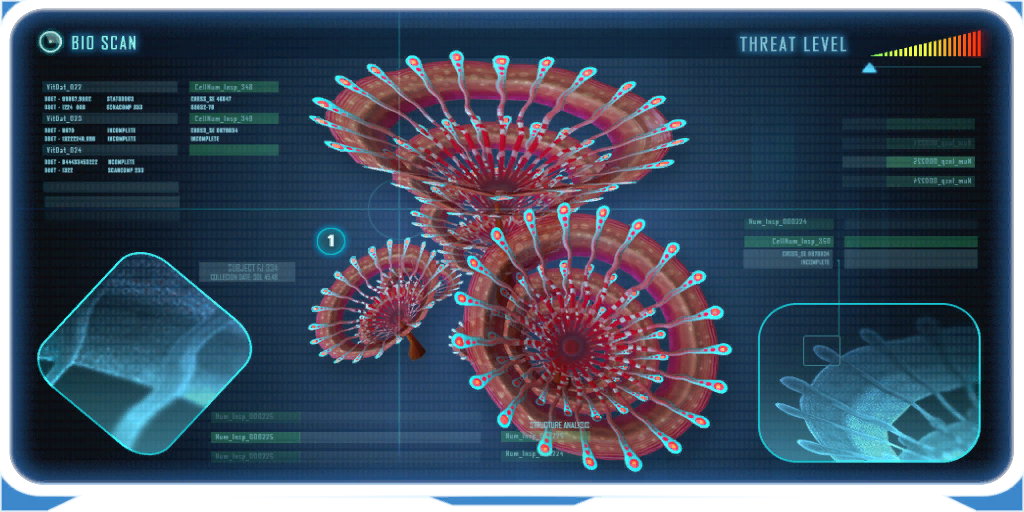
The calculations are done in statistics software, but also standard spreadsheet software has this feature even though lesser flexibility than in statistics software.Īs many other statistical techniques, regression analysis has some assumptions which are particularly relevant if we wish not only to predict data (i.e. This technique is also called the “least squares method”. In general, the best curve will have some property like that the data points are as close as possible to the regression curve in regression analysis this is defined as: the best fit is that of the regression curve for which the sum of the squared distances between data points and regression curve is a minimum. Of course, a criterion must be defined what the “best” curve and the optimal fit is. With the statistical technique of regression analysis, the regression coefficients \(b_0\) and \(b_1\) are estimated such that the regression curve (in this case the straight line) fits best to the given data.
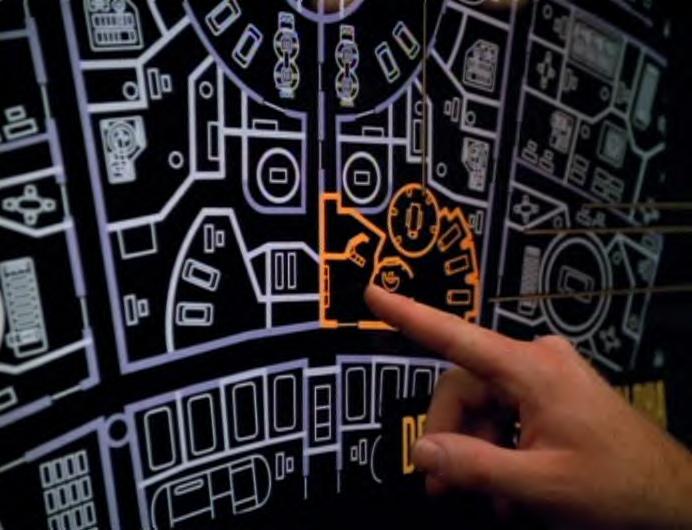
The graphical representation of this model is a straight line (Figure 1). Where \(y\) is the dependent variable and \(x\) the independent variable. We do illustrate the principle of regression for the simple linear regression, that is for the model That means, the prediction read from a regression is rather an estimation than a measurement! One should be clear that the predicted value is, of course, not the true value of the dependent variable of a particular tree, but it should be interpreted as the mean value of all trees with the same value for the independent variable. The variables need to be continuous and on a metric scale. What we look at here is the linear regression which uses mathematical models in which the coefficients are combined by linear operations. The fundamental concept of regression is to establish a statistical relationship between dependent and independent variables such that predictions of the dependent variable can be done. Figure 1 Straight line representing a linear regression model between variable X and Y.
